Tick-Borne Disease
Modeling Tick-Borne Disease Risks in Response to Climate Extremes
Ixodes Ricinus, the most common tick species in Germany and Europe, has been studied extensively for its role as a disease vector. Initial research in the 1930s linked it to tick-borne encephalitis virus (TBEV) and Lyme Borreliosis. Given that Ixodes Ricinus spends around 99% of its 4–6-year lifespan off-host, its survival is closely tied to environmental factors, particularly humidity and temperature. It is an opportunistic feeder, with a wide range of hosts, though deer are among the most common. Relative humidity, vegetation, and other environmental factors contribute significantly to its population dynamics, as these influence the tick’s ability to maintain water balance and increase its questing success.
This study develops a predictive model to assess Ixodes Ricinus proliferation across Germany using seasonal climate forecasts and environmental data. Key variables include temperature, precipitation, solar radiation, humidity, wind speed, vapor pressure, land cover, and elevation. The model utilizes high-resolution climate data downscaled from seasonal forecasts, with variables normalized or transformed to manage outliers and scale differences. Training data were gathered from the Robert Koch Institute and the European Centre for Disease Prevention and Control (ECDC) and span 1990-2022.
Using a neural network model combined with bias correction through random forest regression, we predict tick populations and classify areas into risk categories. Model evaluation was conducted through RMSE for tick abundance predictions and ROC AUC scores for risk classification. The initial RMSE of 0.5 improved to 0.39 after bias correction; however, after reversing the logarithmic transformation, the RMSE increased due to larger deviations in actual tick counts. Spatial analysis revealed that predicted and observed tick distributions are generally aligned, with peak activity observed from March to June, especially in northern and southern Germany, aligning with seasonal environmental conditions favorable to tick activity.
This model provides a valuable tool for understanding tick presence and identifying high-risk areas.
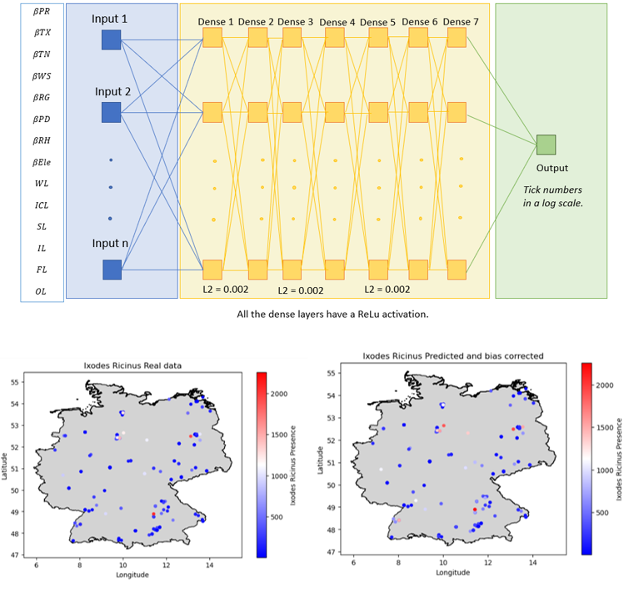
Figure 3. Top: The main structure of the Neural Network used in the model to predict the I. Ricinus presence based on the climatological downscaled data. Bottom: comparison of the predicted and observed values.